Motivations: The Bigger Picture
A series of t-tests were used to explore gender differences while correlations were used to explore age differences and how motivation subcomponents might be related to hours of game play per week.
(Easier-to-interpret graphs on next page.)
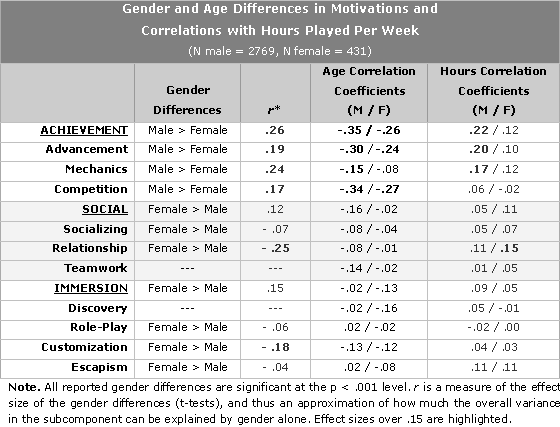
====
Because standardized scores and effect sizes (based on continuous variables) are less-interpretable than percentages (based on categorical variables), a different way of understanding this data is presented here. The "primary motivation" for each player was inferred from their scores. A respondent was assigned a primary motivation if there was no close secondary motivation (primary * .75 > secondary). 57% of players were assigned a primary motivation based on this criteria. This is a somewhat lax criteria but serves the purpose of providing an easier interpretation of the data.
The results from the main components are presented first. Overall, 43% of players did not have a primary motivations using the above-stated criteria. Of those players who did, 20% had Achievement as their primary motivation, 20% had Immersion as their primary motivation, and 17% had the Social component as their primary motivation. The underlying gender and age differences are far more striking when presented using categorical data.
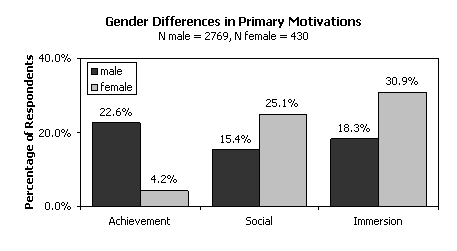
The primary subcomponent was inferred from the largest subcomponent score to preserve enough cases to show the data across the 10 subcomponents.
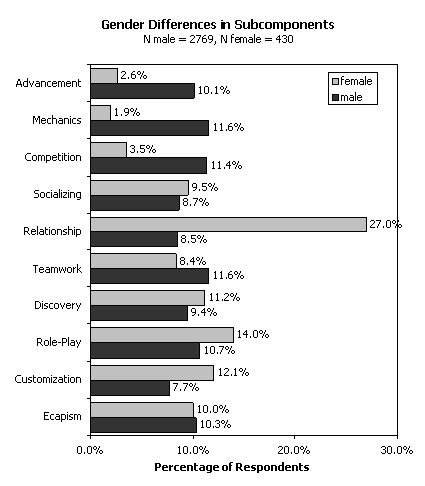
====
Male players tend to be more driven by the Achievement subcomponents than female players, while female players tend to be more driven by the relationship and customization subcomponents. Both male and female players are equally likely to be driven by the socializing, teamwork, discovery, role-playing and escapism subcomponents. Worth noting is that there is a gender difference in the relationship subcomponent but not in the socializing subcomponent although these two subcomponents may seem highly interconnected. In other words, male players socialize just as much as female players but are looking for very different things in those relationships.
Older players are most unlike younger players in the Achievement subcomponents for both male and female players. Younger players are more likely to be driven by advancement, competition and understanding the underlying mechanics. Older players and younger players are not significantly different in terms of the Socializing or Immersion subcomponents.
The best correlates with hours played per week were the advancement and mechanics subcomponents for male players, and the relationship component for female players. In other words, the reasons why men spend a lot of time in the game are different from the reasons why women spend a lot of time in the game. Men who spend a lot of time in the game are using that time to gain levels, get rare items and understand the game mechanics, whereas women who spend a lot of time in the game are using that time to build personal relationships.
The best predictors of hours played per week were explored using a multiple regression with gender, age and the 10 subcomponents as predictors. The model was significant at p < .001 with an adjusted R2 of .06 (not very good model, weak predictors). The best predictor was the advancement subcomponent (Beta = .13, p < .001) followed by the relationship subcomponent (Beta = .10, p < .001). However, none of the subcomponents turned out to be good predictors of hours played per week.
====
The best predictors of problematic usage were explored using a multiple regression with gender, age, hours per week and the 10 subcomponents as factors. A survey scale for problematic usage was developed using the following items (on a fully-labeled 5-point scale using construct-specific response options). These items are based on Ian Danforth's work that teased apart the Engagement factor from the Addiction factor.
• Do you spend more time than you think you should playing the game?
• How difficult would it be for you to limit your playing time?
• How agitated do you get if the servers go down unexpectedly?
• How often do your friends or family members complain about your game play behavior?
• Has your work/school performance suffered because of your game play?
• How much of your happiness in life currently is derived from playing the game?
• Have your personal relationships suffered because of your game-play?
A principal components analysis revealed a single factor with an eigenvalue greater than 1 that accounted for 47% of the overall variance. All items loaded onto this factor with a factor loading between .52 and .79.
The multiple regression was significant at p < .001 with an adjusted R2 of .33 (a good model with strong predictors). The best predictor of problematic usage was the escapism subcomponent (Beta = .31, p < .001), followed by hours played per week (Beta = .27, p < .001) and then the advancement subcomponent (Beta = .18, p < .001).
The results of this multiple regression are interesting in that it shows the escapism subcomponent to be the best predictor of problematic usage. In other words, it is the players who use the online environment as an escape from RL problems that are most likely to develop problematic usage patterns. This is in contrast with claims that it is something inherent in online games that cause addiction and problematic usage. Now, the advancement subcomponent is also a good predictor but not as strong as the escapism subcomponent. The data show that the primary cause of problematic usage are pre-existing RL problems rather than something inherent to online games, and that the game mechanics (often claimed to be strongly addictive) are in fact weaker predictors of problematic usage than the state-of-mind of the player.
====
Respondents also answered (yes / no) whether they had:
- Been on a raid for at least 8 hours
- Ever used a third-party macro or app
- Bought a virtual item / currency
- Ever had an opposite gender character as their main
A series of logistic regressions were used to see which of the 10 subcomponents, in addition to gender and age, might be good predictors for the above 4 statements.
The best predictor for "8 hour raids" was the relationship subcomponent (B = .66, p < .001) followed by the teamwork subcomponent (B = .39, p < .001). While this may seem unintuitive, it does make sense that it is the people who are most interested in serious sustained relationships that would end up in raids of significant duration. And in fact, female players are more likely to have been in an 8 hour raid than male players (35% vs. 26%).
The best predictor for "3rd party macro" was the competition subcomponent (B = .28, p < .001) followed by the mechanics subcomponent (B = .22, p < .001). In other words, third party macros are a way for players to gain an edge over the competition and "optimize" their characters.
The best predictor for "bought virtual item" was age (B = .32, p < .001) followed by the relationship subcomponent (B = .28, p < .001). Age is a significant predictor because of disposable income, but I have no good explanation for why the relationship subcomponent would be a good predictor.
The best predictor for "gender-bending" was the role-playing subcomponent (B = .26, p < .001) followed by the customization subcomponent (B = .24, p < .001).